- Home
- Invited Speakers
Invited Speakers
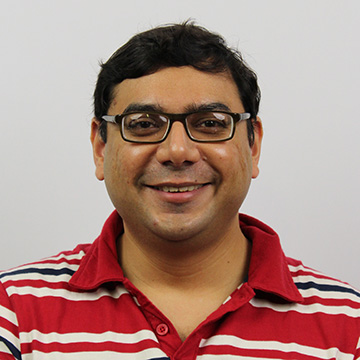
Manik Varma
Partner Researcher, Microsoft Research India
Adjunct Professor, Indian Institute of Technology Delhi
Title: On Extreme Classification & Large Language Models for Search & Recommendation
Manik Varma is a researcher at Microsoft Research India.
Manik received a bachelor's degree in Physics from St. Stephen's College, University of Delhi in 1997 and another one in Computation from the University of Oxford in 2000 on a Rhodes Scholarship.
He then stayed on at Oxford on a University Scholarship and obtained a DPhil in Engineering in 2004.
Before joining Microsoft Research, he was a Post-Doctoral Fellow at the Mathematical Sciences Research Institute Berkeley.
He has been an Adjunct Professor at the Indian Institute of Technology (IIT) Delhi in the Computer Science and Engineering Department since 2009 and jointly in the School of Information Technology since 2011.
His research interests lie in the areas of machine learning, computational advertising and computer vision.
He has served as an (senior) Area Chair for most of the premiere machine learning, artificial intelligence and computer vision conferences such as ACCV, CVPR, ICCV, ICML, ICVGIP and NeurIPS. He is also serving as an associate editor-in-Chief of the IEEE TPAMI journal.
He has been awarded the Government of India's Shanti Swarup Bhatnagar Prize, been elected a Fellow of the Indian Academies of Science (IASc) and Engineering (INAE), awarded Microsoft Gold Star award and has won the PASCAL VOC Object Detection Challenge at ICCV 2009.
Classifiers that he has developed are running live on millions of machines around the world protecting them from viruses and malware.
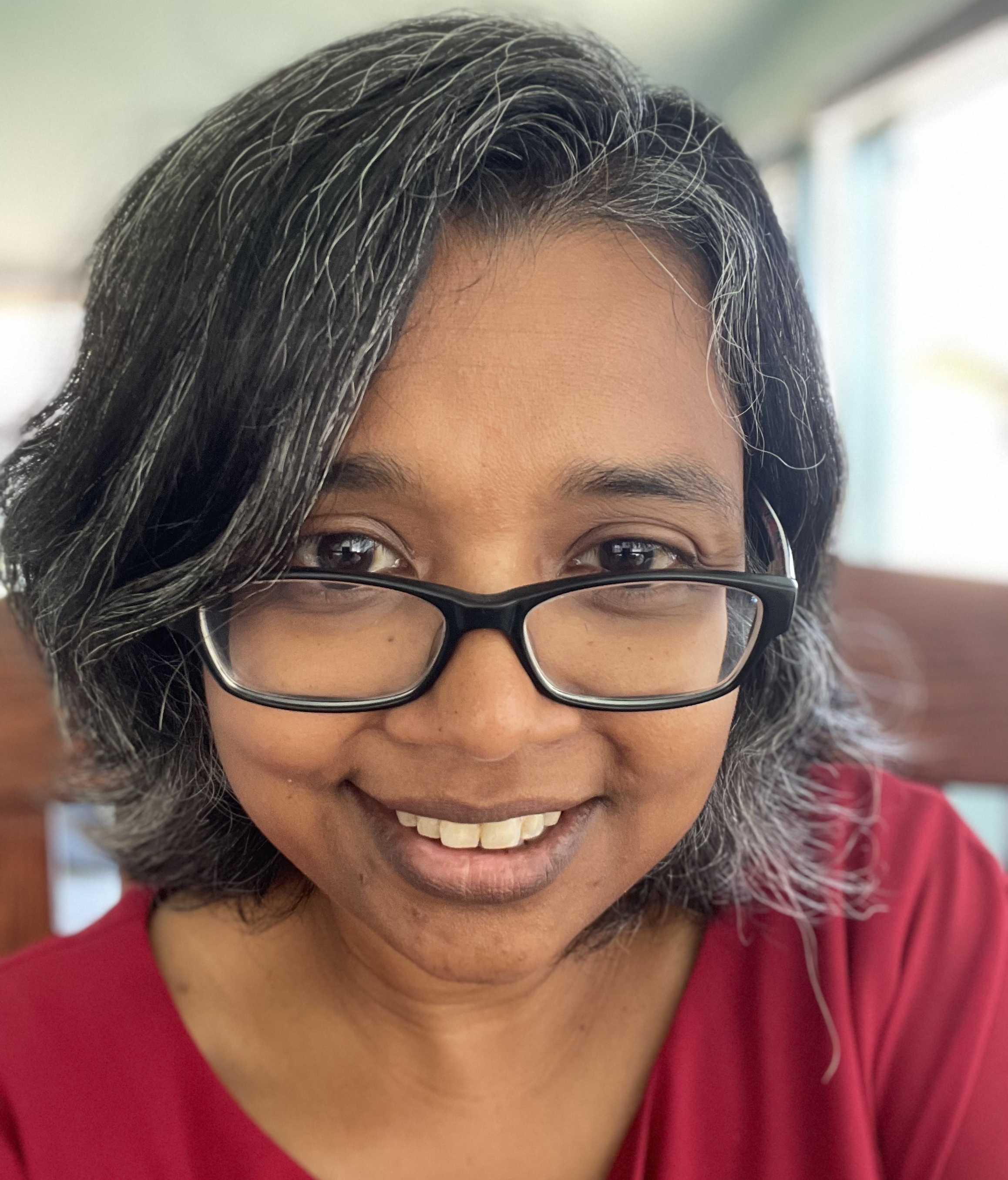
Kamalika Chaudhuri
Research Scientist, Meta AI
Professor, University of California San Diego
Title: Challenges in Privacy-Preserving Machine Learning
Kamalika Chaudhuri received a Bachelor of Technology degree in Computer Science and Engineering in 2002 from Indian Institute of Technology, Kanpur, and a PhD in Computer Science from University of California at Berkeley in 2007. After a postdoc at the Information Theory and Applications Center at UC San Diego, she joined the CSE department at UC San Diego as an assistant professor. She received an NSF CAREER Award in 2013 and a Hellman Faculty Fellowship in 2012.
Kamalika's research interests are in the foundations of trustworthy machine learning, which includes problems such as learning from sensitive data while preserving privacy, learning under sampling bias, and in the presence of an adversary. She is particularly interested in privacy-preserving machine learning, which addresses how to learn good models and predictors from sensitive data, while preserving the privacy of individuals.
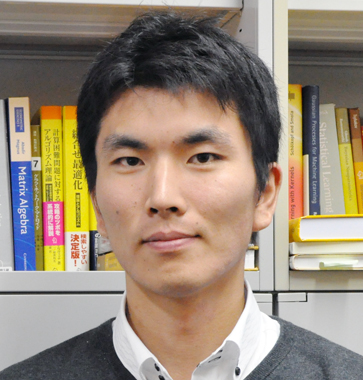
Taiji Suzuki
Associate Professor, The University of Tokyo
Title: Deep learning theory from feature learning perspective
Taiji Suzuki is currently an Associate Professor in the Department of Mathematical Informatics at the University of Tokyo. He also serves as the team leader of “Deep learning theory” team in AIP-RIKEN.
He received his Ph.D. degree in information science and technology from the University of Tokyo in 2009. He has a broad research interest in statistical learning theory on deep learning, kernel methods and sparse estimation, and stochastic optimization for large-scale machine learning problems. He served as area chairs of premier conferences such as NeurIPS, ICML, ICLR, AISTATS and a program chair of ACML.
He received the Outstanding Paper Award at ICLR in 2021, the MEXT Young Scientists’ Prize, Outstanding Achievement Award in 2017 from the Japan Statistical Society, Outstanding Achievement Award in 2016 from the Japan Society for Industrial and Applied Mathematics, and Best Paper Award in 2012 from IBISML.
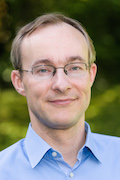
Andreas Krause
Professor of Computer Science, ETH Zurich
Title: Safe and Efficient Exploration via Bayesian World Models
Andreas Krause is a Professor of Computer Science at ETH Zurich, where he leads the Learning & Adaptive Systems Group. He also serves as Academic Co-Director of the Swiss Data Science Center and Chair of the ETH AI Center, and co-founded the ETH spin-off LatticeFlow. Before that he was an Assistant Professor of Computer Science at Caltech. He received his Ph.D. in Computer Science from Carnegie Mellon University (2008) and his Diploma in Computer Science and Mathematics from the Technical University of Munich, Germany (2004).
He is a Max Planck Fellow at the Max Planck Institute for Intelligent Systems, an ELLIS Fellow, a Microsoft Research Faculty Fellow and a Kavli Frontiers Fellow of the US National Academy of Sciences. He received the Rössler Prize, ERC Starting Investigator and ERC Consolidator grants, the German Pattern Recognition Award, an NSF CAREER award, the Okawa Foundation Research Grant recognizing top young researchers in telecommunications as well as the ETH Golden Owl teaching award.
His research on machine learning and adaptive systems has received awards at several premier conferences and journals, including the ACM SIGKDD Test of Time award 2019 and the ICML Test of Time award 2020. Andreas Krause served as Program Co-Chair for ICML 2018, and currently serves as General Chair for ICML 2023 and as Action Editor for the Journal of Machine Learning Research.
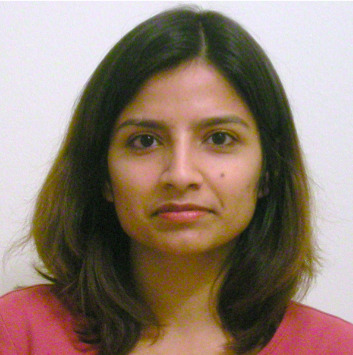
Aarti Singh
Professor, Carnegie Mellon University
Title: Leveraging human factors in sequential decision making
Aarti Singh is a Professor of Machine Learning Department at Carnegie Mellon University.
She was also the recepient of the A. Nico Habermann Career Development Chair award from 2013-2016. She was a Postdoctoral Research Associate at the Program in Applied and Computational Mathematics at Princeton University from 2008-2009 before joining Carnegie Mellon. She received her B.E. in Electronics and Communication Engineering from the University of Delhi in 2001, and M.S. and Ph.D. degrees in Electrical Engineering from the University of Wisconsin-Madison in 2003 and 2008, respectively.
Her research goal is to design algorithms that can learn and leverage inherent structure of data in the form of clusters, graphs, subspaces and manifolds to achieve tradeoffs between the competing goals of computational efficiency and statistical optimality (optimal accuracy for a given amount of data). Additionally, she is investigating how these tradeoffs can be further improved by designing interactive algorithms that employ judicious choice of where, what and how data is acquired, stored and processed. The vision is to introduce a new paradigm of intelligent machine learning algorithms that learn continually via feedback and make high-level decisions in collaboration with humans, thus pushing the envelope of automated scientific and social discoveries. Her research has been supported by grants from NSF, AFOSR, and NIH, including NSF CAREER and the AFOSR Young Investigator Award.