- Program
- Invited Speakers
Invited Speakers
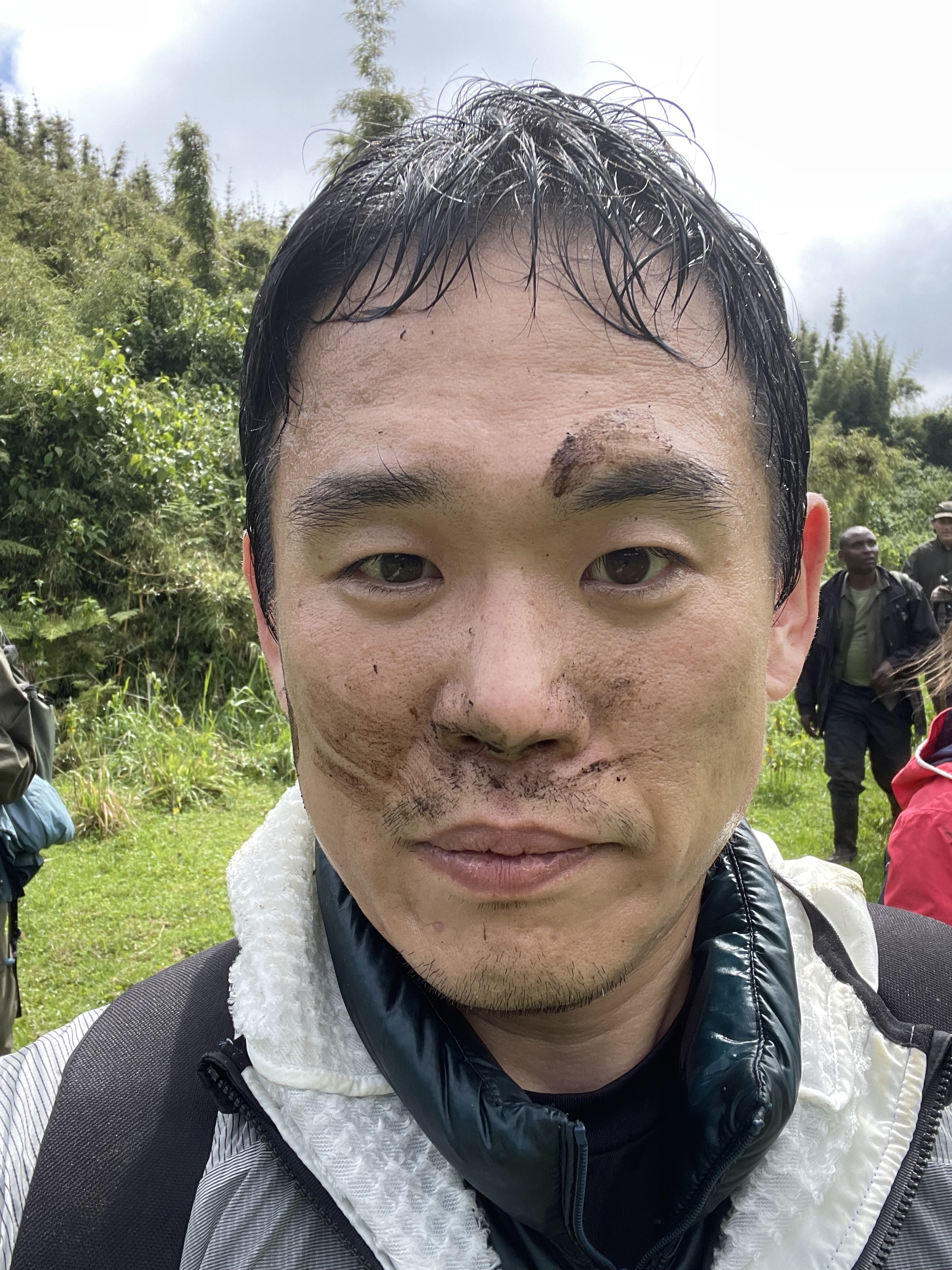
Kyunghyun Cho
Professor, New York University
Title: Beyond Test Accuracies for Studying Deep Neural Networks
Summary: Already in 2015, Leon Bottou discussed the prevalence and end of the training/test experimental paradigm in machine learning. The machine learning community has however continued to stick to this paradigm until now (2023), relying almost entirely and exclusively on the test-set accuracy, which is a rough proxy to the true quality of a machine learning system we want to measure. There are however many aspects in building a machine learning system that require more attention. Specifically, I will discuss three such aspects in this talk; (1) model assumption and construction, (2) optimization and (3) inference. For model assumption and construction, I will discuss our recent work on generative multitask learning and incidental correlation in multimodal learning. For optimization, I will talk about how we can systematically study and investigate learning trajectories. Finally for inference, I will lay out two consistencies that must be satisfied by a large-scale language model and demonstrate that most of the language models do not fully satisfy such consistencies.
Short Bio: Kyunghyun Cho is a professor of computer science and data science at New York University and a senior director of frontier research at the Prescient Design team within Genentech Research & Early Development (gRED). He is also a CIFAR Fellow of Learning in Machines & Brains and an Associate Member of the National Academy of Engineering of Korea. He served as a (co-)Program Chair of ICLR 2020, NeurIPS 2022 and ICML 2022. He is also a founding co-Editor-in-Chief of the Transactions on Machine Learning Research (TMLR). He was a research scientist at Facebook AI Research from June 2017 to May 2020 and a postdoctoral fellow at University of Montreal until Summer 2015 under the supervision of Prof. Yoshua Bengio, after receiving MSc and PhD degrees from Aalto University April 2011 and April 2014, respectively, under the supervision of Prof. Juha Karhunen, Dr. Tapani Raiko and Dr. Alexander Ilin. He received the Samsung Ho-Am Prize in Engineering in 2021. He tries his best to find a balance among machine learning, natural language processing, and life, but almost always fails to do so.
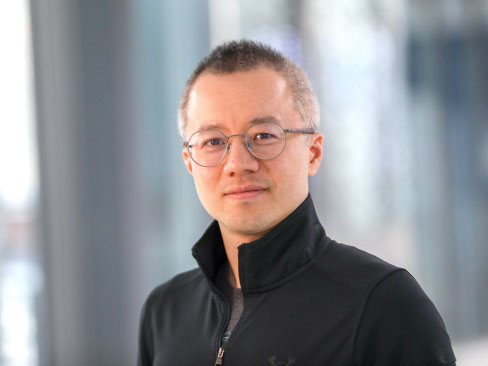
Le Song
Professor, Mohamed bin Zayed University of Artificial Intelligence
Title: Foundation Models for Life Science
Short Bio: Dr. Song is an expert in machine learning and graph neural networks. He is now the CTO and Chief AI Scientist at BioMap, where he leads the efforts in strategic planning and technological development of xTrimo, a family of large-scale foundation models for life science, as well as constructing the high-throughput, closed-looped system to complement the AI engine.
Dr. Song is a professor at MBZUAI, and prior to that, he was an associate professor at the Georgia Institute of Technology, and a researcher at Google and CMU. His work has earned him many best paper awards in major AI conferences such as NeurIPS, ICML and AISTATS to name a few. Dr. Song is also a board member of ICML, and was the program chair for ICML 2022.
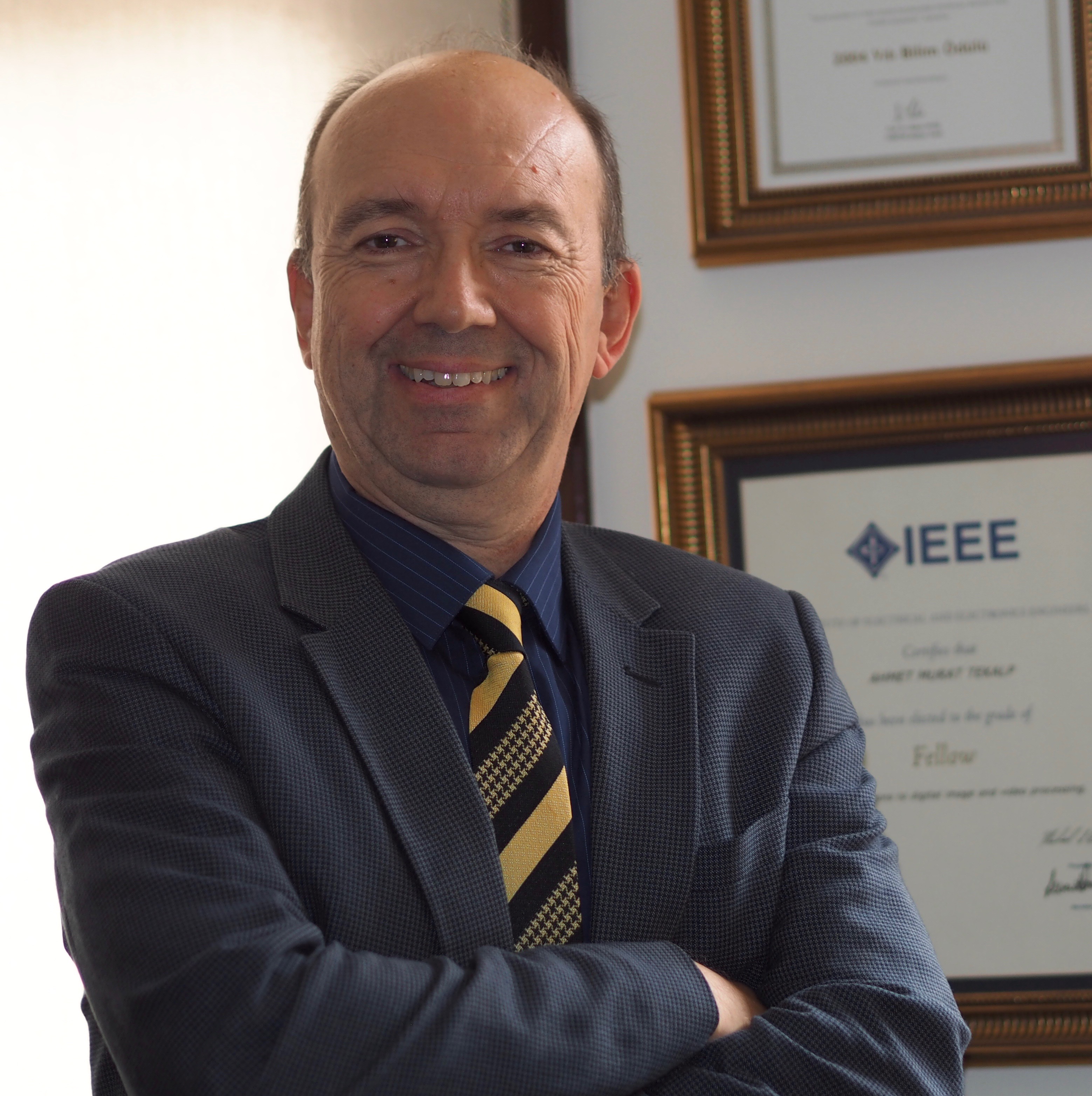
Murat Tekalp
Professor, Koç University
Title: Details or Artifacts: Solving Inverse Problems in Image/Video Processing in the Era of Deep Learning
Summary: Image/video denoising, deburring, super-resolution, and inpainting are examples of ill-posed inverse problems in imaging. The traditional approaches to solving these problems include regression methods, stochastic modeling, sparse modeling, etc. With the advent of deep learning, it is now common to employ nonlinear regression and generative models to solve these ill-posed inverse problems. Yet, one question still remains: How effective are these nonlinear models to separate genuine image details from high-frequency artifacts and hallucinations?
Short Bio: A. Murat Tekalp received Ph.D. in Electrical Computer and Systems Engineering from Rensselaer, Troy, NY (1984). He worked at Eastman Kodak Company (1984-1987), and the University of Rochester (1987-2005), where he was promoted to Distinguished University Professor. He is currently Professor at Koç University, Turkey. He is a Fellow of the IEEE. He was elected to the Turkish Academy of Sciences (2007) and Academia Europaea (2010). He served as an Associate Editor for IEEE Trans. Signal Processing (1990-1992) and IEEE Trans. Image Processing (1994-1996). He was the Editor-in-Chief of Signal Processing: Image Communication (Elsevier) from 1999 to 2010. He chaired IEEE Image and Multidimensional Signal Processing Technical Committee (Jan.'1996-Dec.'1997), and was the General Chair of IEEE ICIP (2002). He served on the Editorial Boards of IEEE Signal Processing Magazine (2007-2010) and Proceedings of the IEEE (2014-2019). He is currently on the Editorial Board of Wiley-IEEE Press. He authored Digital Video Processing, Prentice Hall (1995, 2015). Dr. Tekalp holds 9 US patents.
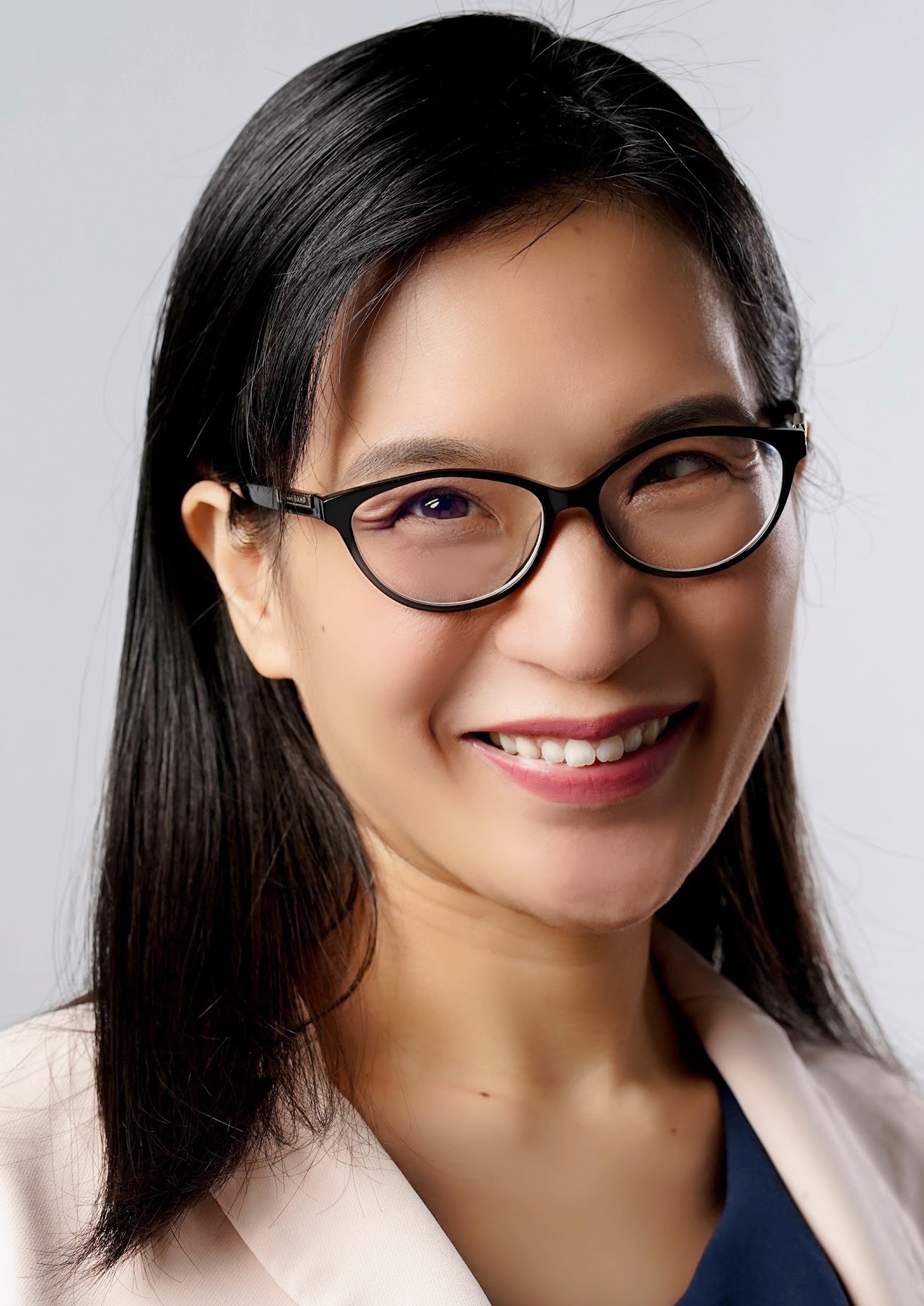
Nancy F. Chen
Investigator at CFAR (Centre for Frontier AI Research), A*STAR
Title: SeaEval for Multilingual Foundation Models: From Cross-Lingual Alignment to Cultural Reasoning
Summary: We present SeaEval, a benchmark for multilingual foundation models. In addition to characterizing how these models understand and reason with natural language, we also investigate how well they comprehend cultural practices, nuances, and values. Alongside standard accuracy metrics, we examine the brittleness of foundation models in the dimensions of semantics and multilinguality. Our investigations encompasses both open-source and proprietary models, shedding light on their behavior in classic NLP tasks, reasoning, and cultural contexts. Notably, (1) Most models respond inconsistently to paraphrased instructions. (2) Exposure bias pervades, evident in both standard NLP tasks and cultural understanding. (3) For questions rooted in factual, scientific, or common sense knowledge, consistent responses are expected across multilingual queries that are semantically equivalent. Yet, many models intriguingly demonstrate inconsistent performance on such queries. (4) Models trained multilingually still lack ``balanced multilingual'' capabilities. Our endeavors underscore the need for more generalizable semantic representations and enhanced multilingual contextualization. SeaEval can serve as a launchpad for in-depth investigations for multilingual and multicultural evaluations.
Short Bio: Nancy F. Chen is an A*STAR fellow, senior principal scientist, principal investigator, and group leader at I2R (Institute for Infocomm Research) and Principal Investigator at CFAR (Centre for Frontier AI Research). Her group works on generative AI in speech, language, and conversational technology. Her research has been applied to education, defense, healthcare, and media/journalism. Dr. Chen has published 100+ papers and supervised 100+ students/staff. She has won awards from IEEE, Microsoft, NIH, P&G, UNESCO, L’Oréal, SIGDIAL, APSIPA, MICCAI. She is an IEEE SPS Distinguished Lecturer (2023-2024), Program Chair of ICLR 2023, Board Member of ISCA (2021-2025), and Singapore 100 Women in Tech (2021). Technology from her team has led to commercial spin-offs and government deployment. Prior to A*STAR, she worked at MIT Lincoln Lab while doing a PhD at MIT and Harvard.