Speakers
Keynote Speakers
Bernhard Schölkopf, Professor and Director of Max Planck Institute for Intelligent Systems, Germany
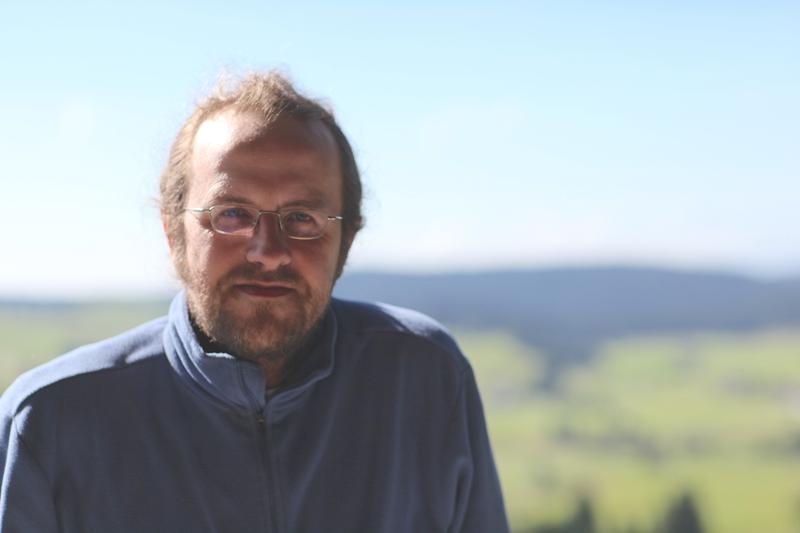
"Causal Learning"
In machine learning, we use data to automatically find dependences in the world, with the goal of predicting future observations. Most machine learning methods build on statistics, but one can also try to go beyond this, assaying causal structures underlying statistical dependences. Can such causal knowledge help prediction in machine learning tasks? We argue that this is indeed the case, due to the fact that causal models are more robust to changes that occur in real world datasets. We discuss implications of causality for machine learning tasks, and argue that many of the hard issues benefit from the causal viewpoint. This includes domain adaptation, semi-supervised learning, transfer, life-long learning, and fairness, as well as an application to the removal of systematic errors in astronomical problems.
Bernhard Schölkopf's scientific interests are in machine learning and causal inference. He has applied his methods to a number of different application areas, ranging from biomedical problems to computational photography and astronomy. Bernhard has researched at AT&T Bell Labs, at GMD FIRST, Berlin, and at Microsoft Research Cambridge, UK, before becoming a Max Planck director in 2001. He is a member of the German Academy of Sciences (Leopoldina), and has received the J.K. Aggarwal Prize of the International Association for Pattern Recognition, the Max Planck Research Award (shared with S. Thrun), the Academy Prize of the Berlin-Brandenburg Academy of Sciences and Humanities, and the Royal Society Milner Award.
Tom Dietterich, Distinguished Professor (Emeritus) and Director of Intelligent Systems, Oregon State University, USA
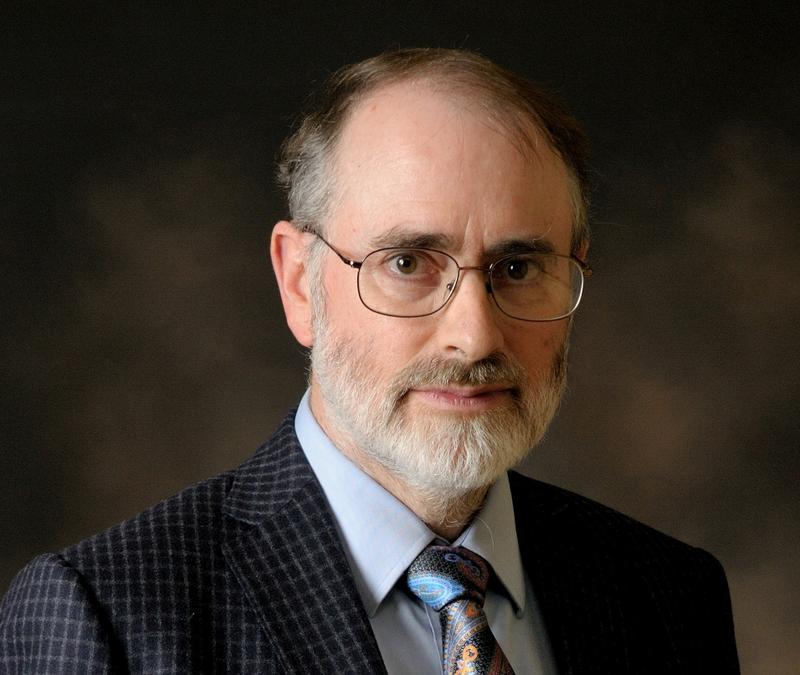
"Combining AI and Visualization to Manage Ecosystems"
As humans occupy virtually all of the planet, we must actively manage ecosystems in order to ensure their sustained functioning. Many ecosystem problems can be formulated as Markov Decision Problems (MDPs) in which the state transitions are provided by a simulator rather than by an explicit function. The two key technical challenges in solving such MDPs are (a) the state spaces are immense and (b) the simulators are very expensive. A third, more political, challenge (c) is that ecosystem management problems involve many stakeholders who often disagree about the objectives to be optimized. To address the first problem, we employ search in a parameterized policy space. We have obtained excellent results using SMAC, a form of Bayesian Optimization, to find good policies. To address the second problem, we extend the method of Model-Free Monte Carlo (MFMC) to create a surrogate model. We reduce the size of the state space by factoring out exogeneous state variables. To address the third problem, we have built a visualization environment, MDPvis, that allows multiple stakeholders to modify the MDP reward function and explore the behavior of the system. We hope that the combination of visualization and rapid MDP solution will help multiple stakeholders arrive at consensus on how to manage complex ecosystems.
Dr. Dietterich (AB Oberlin College 1977; MS University of Illinois 1979; PhD Stanford University 1984) is Professor Emeritus and Director of Intelligent Systems Research in the School of Electrical Engineering and Computer Science at Oregon State University, where he joined the faculty in 1985. Dietterich is one of the pioneers of the field of Machine Learning and has authored more than 130 refereed publications and two books. His research is motivated by challenging real world problems with a special focus on ecological science, ecosystem management, and sustainable development. He is best known for his work on ensemble methods in machine learning including the development of error-correcting output coding. Dietterich has also invented the MAXQ decomposition for hierarchical reinforcement learning.
Dietterich has devoted many years of service to the research community. He is Past President of the Association for the Advancement of Artificial Intelligence, and he previously served as the founding president of the International Machine Learning Society. Other major roles include Executive Editor of the journal Machine Learning, co-founder of the Journal for Machine Learning Research, and program chair of AAAI 1990 and NIPS 2000. Dietterich is a Fellow of the ACM, AAAI, and AAAS.
Invited Speakers
Hang Li, Toutiao, China
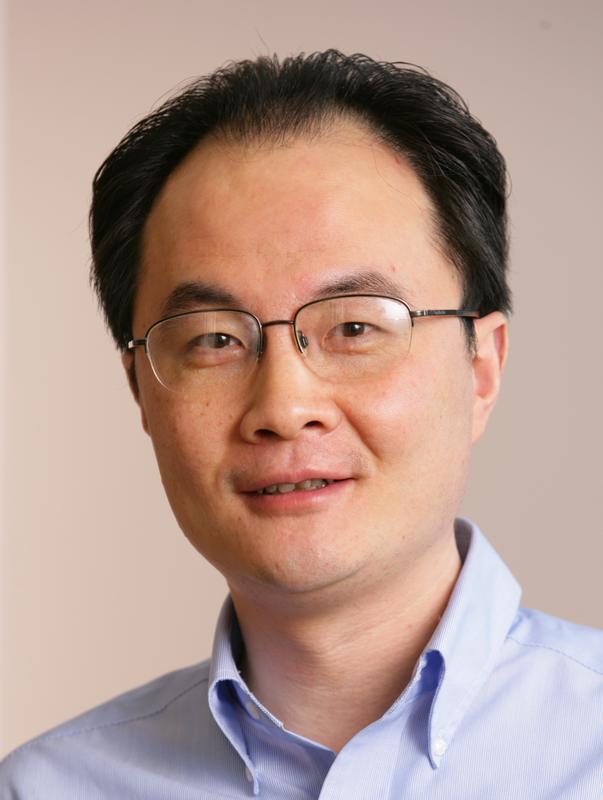
"Beyond Deep Learning: Combining Neural Processing and Symbolic Processing"
Recently deep learning has brought significant breakthroughs to natural language processing. I will start the talk by summarizing the strengths and limitations of deep learning in natural language processing. I will then indicate that a hybrid approach combining neural processing (deep learning) and symbolic processing would be necessary and even more powerful for the tasks in natural language processing, particularly question answering. This is because the two paradigms are both advantageous and complementary; symbolic representations are easier to interpret and manipulate, while neural representations are more suitable for dealing with uncertainty in language and noise in data. Finally, I will introduce our recent effort toward developing neural symbolic processing, including building of a hybrid system for question answering from relational database and a hybrid system for generative question answering from knowledge base.
Hang Li is director of Toutiao AI Lab, adjunct professors of Peking University and Nanjing University. He is an IEEE Fellow and an ACM Distinguished Scientist. His research areas include information retrieval, natural language processing, machine learning, and data mining. Hang graduated from Kyoto University in 1988 and earned his PhD from the University of Tokyo in 1998. He worked at NEC Research as researcher from 1990 to 2001, Microsoft Research Asia as senior researcher and research manager from 2001 to 2012, and chief scientist and director of Huawei Noah’s Ark from 2012 to 2017. He joined Toutiao in 2017. Hang has published three technical books, and more than 120 technical papers at top international conferences including SIGIR, WWW, WSDM, ACL, EMNLP, ICML, NIPS, SIGKDD, AAAI, IJCAI, and top international journals including CL, NLE, JMLR, TOIS, IRJ, IPM, TKDE, TWEB, TIST. He and his colleagues’ papers received the SIGKDD’08 best application paper award, the SIGIR’08 best student paper award, the ACL’12 best student paper award. Hang worked on the development of several products such as Microsoft SQL Server 2005, Office 2007, Live Search 2008, Bing 2009, Office 2010, Bing 2010, Office 2012, Huawei smartphones 2014 and Huawei smartphones 2017. He has 42 granted US patents. Hang is also very active in the research communities and has served or is serving top international conferences as PC chair, Senior PC member, or PC member, including SIGIR, WWW, WSDM, ACL, NACL, EMNLP, NIPS, SIGKDD, ICDM, IJCAI, ACML, and top international journals as associate editor or editorial board member, including CL, IRJ, TIST, JASIST, JCST.
Eunho Yang, Assistant Professor in the School of Computing at KAIST, Korea
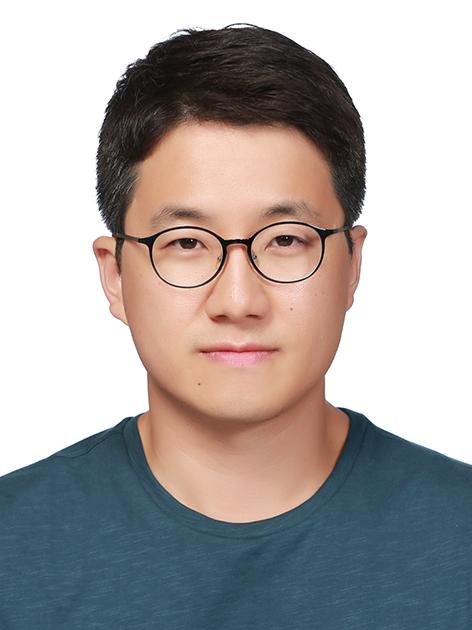
"Beyond Gaussian/Ising Graphical Models"
Graphical models are the standard toolkit to model interactions between huge number of multiple random variables. Gaussian graphical models for continuous data and Ising (or discrete graphical models) for discrete data are the two most popular instances of undirected graphical models. In this talk, we will discuss limitations of these popular instances of pairwise graphical models with respect to two orthogonal directions: i) restriction on types of data following bell-shpaed Gaussian or categorical properties ii) lack of higher-order interactions due to computational overheads. We will then introduce more general graphical models beyond Gaussian and Ising graphical models, to overcome these limitations.
Eunho Yang is an assistant professor at the School of Computing, KAIST. Before joining KAIST, he spent two years at IBM T.J. Watson Research Center as a Research Staff Member. He obtained his Ph.D. in 2014 from the university of Texas at Austin, and did M.S. and B.S from the Seoul National University, Korea in 2006 and 2004, respectively. His research interests are in statistical machine learning in general with the special focuses on high-dimensional statistics. He is currently developing new theories and algorithms for graphical models and deep learning, with the applications of computational biology and medicine, etc.