KEYNOTE SPEAKERS
Prof. Heng Ji
University of Illinois Urbana-Champaign
Title: Making Large Language Model’s Knowledge More Accurate, Organized, Up-to-date and Fair
Large language models (LLMs) have demonstrated remarkable performance on knowledge reasoning tasks, owing to their implicit knowledge derived from extensive pretraining data. However, their inherent knowledge bases often suffer from disorganization and illusion, bias towards familiar entities, and rapid obsolescence. Consequently, LLMs frequently make up untruthful information, exhibit resistance to updating outdated knowledge, or struggle with generalizing across multiple languages. In this talk, Heng Ji will aim to answer the following questions:
- Where and How is Knowledge Stored in LLM?
- Why does LLM Lie?
- How to Update LLM’s Dynamic Knowledge?
- How can we reach LLM’s Knowledge Updating Ripple Effect?
- What can knowledge + LLM do for us?
She will also present a case study on “SmartBook” – situation report generation.

Prof. Yisong Yue
California Institute of Technology
Title: Neurosymbolic AI for Safety-Critical Agile Control
This talk overviews research at Caltech on designing hybrid or neurosymbolic AI systems that blend learning with symbolic structure to achieve both the flexibility of the former and the formal interpretability and generalization power of the latter. By having formally interpretable systems, one can employ a wide range of formal analysis techniques to verify essential properties of the overall system, such as those related to safety and stability, and use those analyses to guide system design and optimization.
Focusing on formally interpretable structure arising from control and planning, he will present new algorithms and their deployment in a range of applications, including agile flight control under challenging and time-varying environments, controlling highly underactuated systems (e.g., one-legged hoppers), as well as briefly overview other related research.

Dr. Thang Luong
Google Deepmind
Title: AI Superhuman Reasoning for Math and beyond
In his talk, Dr. Thang Luong will discuss the recent advances in AI for Math and beyond. Through the talk, he will also share his perspective on the future of AI and hint towards a bigger picture of advancing the reasoning capabilities of existing AI systems.
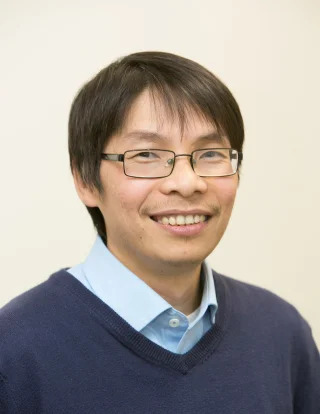
Prof. Dinh Phung
Monash University
Title: Learning as Distribution Matching: A Perspective through Optimal Transport
The author will address the problem of distribution matching as an emerging approach to various learning tasks. This includes a discussion on statistical divergences, highlighting their desirable properties and limitations, which motivates an exploration of optimal transport and the Wasserstein distance. A brief historical overview will be provided, with a focus on the properties of these concepts and their potential applications in diverse machine learning tasks. The discussion will conclude with an examination of how these tools can be specifically employed to tackle important tasks in areas such as robust machine learning, generative modeling, domain transfer, and graphical models.