Keynote Speaker
Clustering - what both Theoreticians and Practitioners are Doing Wrong
Speaker: Prof. Shai Ben-David, University of Waterloo
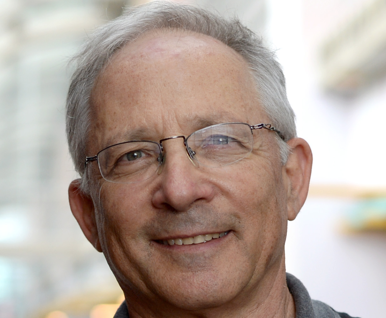
Shai Ben-David grew up in Jerusalem, Israel. He attended the Hebrew University studying mathematics, physics and psychology and received his PhD for a thesis in set theory. After a post doctorate at the University of Toronto he joined the CS Department at the Technion (Israel Institute of Technology). In August 2004 he joined the School of Computer Science at the University of Waterloo - his current affiliation. Over the years, Prof Ben-David has held visiting faculty positions at the Australian National University, Cornell University, ETH Zurich, TTI Chicago and the Simons institute at Berkeley. Prof. Ben-David has served as a program chair for the major machine learning theory conferences (COLT and ALT, and area chair for ICML, NIPS and AISTATS). He has co-authored the textbook "Understanding machine learning" and well over a hundred research papers on ML theory, computational complexity and logic.
Abstract
Unsupervised learning is widely recognized as one of the most important challenges facing machine learning nowadays. However, in spite of hundreds of papers on the topic being published every year, current theoretical understanding and practical implementations of such tasks, in particular of clustering, is very rudimentary. My talk focuses on clustering. I claim that the most significant challenge for clustering is model selection. In contrast with other common computational tasks, for clustering, different algorithms often yield drastically different outcomes. Therefore, the choice of a clustering algorithm, and their parameters (like the number of clusters) may play a crucial role in the usefulness of an output clustering solution. However, currently there exists no methodical guidance for clustering tool-selection for a given clustering task. Practitioners pick the algorithms they use without awareness to the implications of their choices, and the vast majority of theory of clustering papers focuses on analyzing the resources (time, sample sizes etc) needed to solve optimization problems that arise from picking some concrete clustering objective. However, the benefits of picking an efficient algorithm for a given objective pale in comparison to the costs of mismatch between the clustering objective picked and the intended use of clustering results. I will argue the severity of this problem and describe some recent proposals aiming to address this crucial lacuna.
Machine Learning in Autonomous Systems: Theory and Practice
Speaker: Prof. Daniel D. Lee, Cornell Tech & Samsung Research
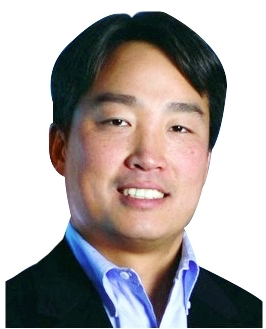
Dr. Daniel D. Lee is currently Professor in Electrical and Computer Engineering at Cornell Tech and Executive Vice President for Samsung Research. He previously was the UPS Foundation Chair Professor in the School of Engineering and Applied Science at the University of Pennsylvania. He received his B.A. summa cum laude in Physics from Harvard University and his Ph.D. in Condensed Matter Physics from the Massachusetts Institute of Technology in 1995. After completing his studies, he was a researcher at AT&T and Lucent Bell Laboratories in the Theoretical Physics and Biological Computation departments. He is a Fellow of the IEEE and AAAI and has received the National Science Foundation CAREER award and the Lindback award for distinguished teaching. He was also a fellow of the Hebrew University Institute of Advanced Studies in Jerusalem, an affiliate of the Korea Advanced Institute of Science and Technology, and organized the US-Japan National Academy of Engineering Frontiers of Engineering symposium and Neural Information Processing Systems (NIPS) conference. His research focuses on understanding general computational principles in biological systems, and on applying that knowledge to build intelligent robotic systems that can learn from experience.
Abstract
Current artificial intelligence (AI) systems for perception and action incorporate a number of techniques: optimal observer models, Bayesian filtering, probabilistic mapping, trajectory planning, dynamic navigation and feedback control. I will briefly describe and demonstrate some of these methods for autonomous driving and for legged and flying robots. In order to model data variability due to pose, illumination, and background changes, low-dimensional manifold representations have long been used in machine learning. But how well can such manifolds be processed by neural networks? I will highlight the role of neural representations and discuss differences between synthetic and biological approaches to computation and learning.
Invited Speaker
Something Old, Something New, Something Borrowed, ...
Speaker: Prof. Wray Buntine, Monash University
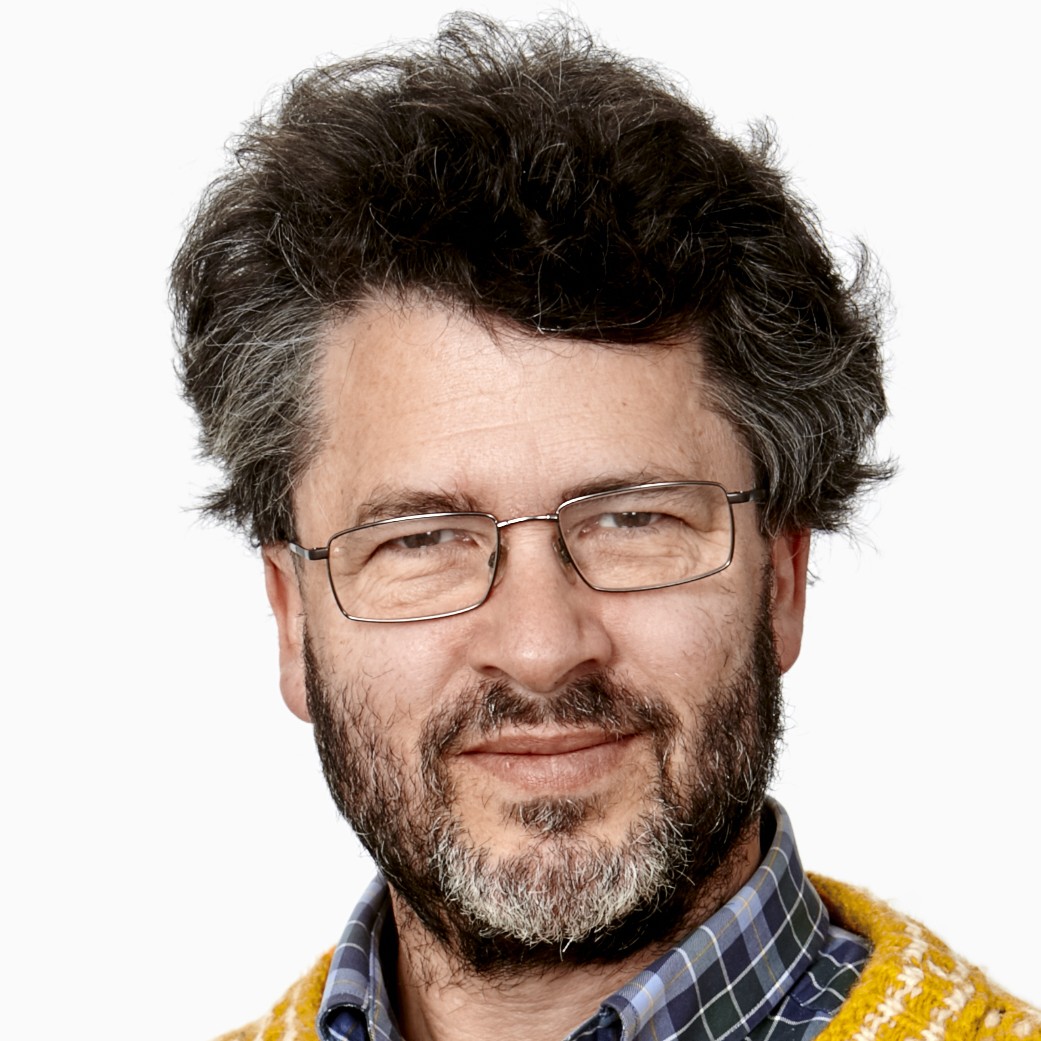
Wray Buntine is a full professor at Monash University from 2014 and is director of the Master of Data Science, the Faculty of IT's newest and in-demand degree. He was previously at NICTA Canberra, Helsinki Institute for Information Technology where he ran a semantic search project, NASA Ames Research Center, University of California, Berkeley, and Google. He is known for his theoretical and applied work and in probabilistic methods for document and text analysis, social networks, data mining and machine learning.
Abstract
Something Old: In this talk I will first describe some of our recent work with hierarchical probabilistic models that are not deep neural networks. Nevertheless, these are currently among the state of the art in classification and in topic modelling: k-dependence Bayesian networks and hierarchical topic models, respectively, and both are deep models in a different sense. These represent some of the leading edge machine learning technology prior to the advent of deep neural networks. Something New: On deep neural networks, I will describe as a point of comparison some of the state of the art applications I am familiar with: multi-task learning, document classification, and learning to learn. These build on the RNNs widely used in semi-structured learning. The old and the new are remarkably different. So what are the new capabilities deep neural networks have yielded? Do we even need the old technology? What can we do next? Something Borrowed: to complete the story, I'll introduce some efforts to combine the two approaches, borrowing from earlier work in statistics.
AI for Transportation
Speaker: Dr. Jieping Ye, Didi AI Labs & University of Michigan
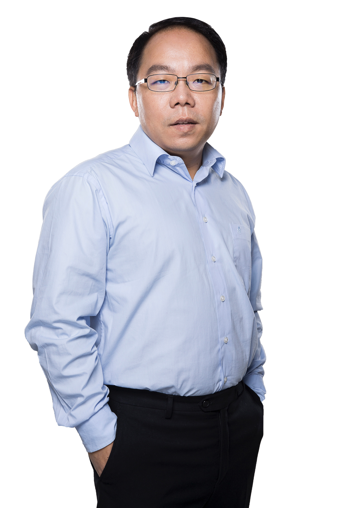
Dr. Jieping Ye is head of Didi AI Labs, a VP of Didi Chuxing and a Didi Fellow. He is also an associate professor of University of Michigan, Ann Arbor. His research interests include big data, machine learning, and data mining with applications in transportation and biomedicine. He has served as a Senior Program Committee/Area Chair/Program Committee Vice Chair of many conferences including NIPS, ICML, KDD, IJCAI, ICDM, and SDM. He has served as an Associate Editor of Data Mining and Knowledge Discovery, IEEE Transactions on Knowledge and Data Engineering, and IEEE Transactions on Pattern Analysis and Machine Intelligence. He won the NSF CAREER Award in 2010. His papers have been selected for the outstanding student paper at ICML in 2004, the KDD best research paper runner up in 2013, and the KDD best student paper award in 2014.
Abstract
Didi Chuxing is the world’s leading mobile transportation platform that offers a full range of app-based transportation options for 550 million users. Every day, DiDi's platform receives over 100TB new data, processes more than 40 billion routing requests, and acquires over 15 billion location points. In this talk, I will show how AI technologies have been applied to analyze such big transportation data to improve the travel experience for millions of users.